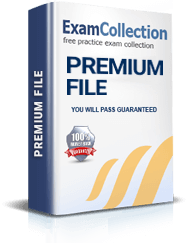
100% Real Microsoft Data Science DP-100 Exam Questions & Answers, Accurate & Verified By IT Experts
Instant Download, Free Fast Updates, 99.6% Pass Rate
DP-100 Premium File: 562 Questions & Answers
Last Update: Mar 21, 2025
DP-100 Training Course: 80 Video Lectures
DP-100 PDF Study Guide: 608 Pages
$79.99
Microsoft Data Science DP-100 Practice Test Questions in VCE Format
Microsoft Data Science DP-100 Practice Test Questions, Exam Dumps
Microsoft DP-100 Designing and Implementing a Data Science Solution on Azure exam dumps vce, practice test questions, study guide & video training course to study and pass quickly and easily. Microsoft DP-100 Designing and Implementing a Data Science Solution on Azure exam dumps & practice test questions and answers. You need avanset vce exam simulator in order to study the Microsoft Data Science DP-100 certification exam dumps & Microsoft Data Science DP-100 practice test questions in vce format.
Hello and welcome to the Data Processing with Azure ML section. In the previous lecture, we learned about how to upload a data set to Azure MLWorkspace and how to enter the data manually. In this lecture, we will cover how to convert the data set format as well as how to unpack the zip data set. So let's go to the Azure ML Studio and see how we can convert the CSV file into a TSV file and vice versa. All right, there we are in our Azure ML Studio. We have this CSV file right here that we uploaded in the previous lecture. Let's drag and drop it here. And let's now go to the data format conversion. As we all know by now, all that we need to do is drag and drop this module, called Convert to TSV, onto the canvas. In order for us to process the CSV, all we need to do is simply attach this output node from the CSV dataset to the input node of the Convert to CSV module. After we have done this, we need to run this particular module. So let's click on Run to execute this module. It's running. And please allow me to pause the video while it runs and return when it is finished. All right, Azure has now converted the existing CSV into a TSV file. It has run successfully. Similarly, we can apply other data format conversions, and I suggest you try those also during your free time. At the same time, you can download this by right clicking on this note here and clicking on "Download" to check the output. All right, let's now go ahead and learn some other modules that are available. Let me just delete these things so that we have some space on the canvas. But before I delete these, let me also show you what the various UI components of this particular canvas are, as well as the Studio. We have covered some of these earlier. However, some of the UI elements have not been covered, such as the minimap. Minimap allows you to have a quick preview of the entire experiment. You can always show or hide the minimap. You also have these zoom-in and zoom-out buttons. You can zoom into the actual size, or you can zoom in to fit. You also have this Run History button, which will show you how many times this particular experiment was run. What is the name, the state, and the status of it—if it is finished or gives any particular error or not? Now, the start and end times of that particular experiment can also be viewed from here. Okay, so by clicking on Go back to the experiment, we can go back to our Canvas. And what did we want to do? We wanted to delete these two free spaces. So we can simply select these modules and press the Delete button to delete them. That's what I love about azure. The user experience has been very consistent with what we are already used to. Okay, now let me talk about the unpack zipped data set module, which can be pretty handy to save time while uploading the large data sets. You can simply zip them locally and then upload them in order to unpack them using the Studio. Depending on the speed, this could save some time. All right, I already have a zipdata set on my local drive. This was originally a CSV file that has been compressed and zipped. Now that we know how to upload a data set, we click on "New" then "Data Set," and let's try to upload our zip file from the local files. Choose the file. Let's upload this to our workspace. It's uploading. Let me pause this and come back to you once it has finished uploading. Great. It has now uploaded the zip file, and let's now try to unpack our zip data set. So we click on Save data set, My Data Sets, and then drag and drop our zip data set onto the canvas. You can see some basic information on the right-hand side here. All right, so what was the name of the module that we were going to use? It was unpacked. zip data set. Great. But now I may have to browse and scroll up and down to use this module in my experiment. And if there are so many options available, it can be pretty difficult. However, there is this really simple but very useful feature of searching for modules and functions. You can simply type the name of the module you are looking for, such as unpacked. There you go. And we can then drag and drop the unpacked ZIP data set onto the experiment. As you can see, there are two nodes here, one accepting the input and the other giving an output. In our case, we are trying to unpack a zipped dataset, so the input to it will be the zip dataset and the output should be an unpacked CSV file. Let's just connect these nodes, and now we are good to run it and see the output. So let me click on run; it's running; and let me come back to you after it has finished running. Wow, it has run successfully, and now we are ready to visualise the output. So I go to the node, right-click Visualize, and there you go. Well, here is the output. But what just happened here? While it has successfully unpacked the data set, our header row is now part of the data points, and the header has some generic names such as call one, call two, and so on. Now let's go back to the parameters of this module and check why it could have happened. Well, there is the problem. As you can see, among various other parameters, we also have this checkbox here that says "File has header row." Our CSV file does have a header rule. So let's check this box and run it again to see if it has run successfully. And now let's check the output by clicking on the output note and clicking on visualize. And there you go. Now our data set is uploaded correctly. Very good. This is the end of the lecture on data input and output to Azure Workspace. In this lecture, we have seen how to convert the data formats as well as how to unpack a zip data set. In the next lecture, we will see how to import the data from an external source. the next class. And thank you so much for joining me in this lecture. Have a great time ahead.
Hello and welcome. In the previous lectures, we learned about how to upload the data and how to convert and unpack a Zipped data set. However, we may not always have our data in a CSV or PSV file. You may not want to take the pain of downloading the data set from some website or from some other storage, formatting it, and then uploading it to Azure. You may want to obtain the data directly from an external source. So then the question is, how do we import the data from other sources? Let's go to the Azure ML Studio and take a look at the Import Data module. Here we are. Let's search for the import data module. I'm going to type "Import" and drag and drop it onto the canvas. Import Data Module imports data from Azure Blob Storage, Hive Queryweb URL via http, Azure Tables, Azure SQL Database, and Azure Document DB. Let's try to import the data using WebURL via the HTTP method, which I believe all of us would be able to complete. All the other methods are equally simple, as long as you have the required credentials to access the data. So we select the Web URL via http://ittasks.us for the source URL, and what is the source data format along with a couple of checkboxes? The first check box is to tell Azurao whether the source data has hit a row or not. The second checkbox is very interesting. Let me explain that. First, let's say you have run the Import data module once and now want to run the experiment again. If we run the Import Data Module also, we may end up running it every time the experiment is run. By ticking this check box, Azure will use the results from the cache and not make the import request from the URL all the time. All right, let's go ahead and import the dataset from this URL that I have already copied. Okay, let's go to the URL first and have a look at our data. I have already provided the URL in the text file as part of the course files. Let me paste this URL and hit Enter. And here is our data set. So this is the data that we would like to import for an experiment. There are two things we need to do to configure this. By pasting the URL in the data source URL field and giving the rest of the parameters, we know that the data is delimited. We can also see that the dataset does not have any header information. So we uncheck the check box that says CSV or TSV has hit a row. Now let's select the module and run it. I'm going to pause the video and come back once it has run successfully. It has run successfully, and it's time to visualise the data. So I right-click on this output node and click to visualize. It may take some time as the data set is huge. There you go. There is our data imported from the URL, ready for further processing if needed. Let me also show you how the exact same results can be achieved by using the Import Wizard. All right, let me close this so we can select the Import Data module. Click on "Launch." The Import Data Wizard It will open this pop-up with multiple options, which are the same as the drop-down options that we saw earlier. Select the web address via HTTP and click on the forward arrow. Test the URL and select the data format along with the appropriate tick. and it gives you a message that it has been successfully configured. It doesn't really add too much value, but some may find one method more useful than the other. and you can run that. I'm not going to run it now because the results are going to be the same. That basically concludes this lecture on the input and output of data to Azure Workspace. In this lecture, we have seen how to import the data from an external source using the UCI Adult Census data. In the next few lectures, we will see some more modules that deal with adding columns and rows, applying SQL transformations on the data, removing duplicate rows, and selecting columns of interest. We will also deal with editing the metadata, such as converting the column format from numeric to string and string to numeric and vice versa, as well as cleaning the missing data. So I'll see you in the next class, and thank you so much for joining me in this one. Have a great day. Time.
Hello and welcome. In the last lecture, we uploaded a sample data set and also covered how we can upload various types of data sets such as CSV, TSV, and zip files. We also unpacked a ZIP data set using the unpack Zip data set model. We not only entered the data manually but also imported one data set using an UCI link for this data set. Today, we are going to cover part of the data transformation. Today we will cover some of the modules in data manipulation, namely Add Columns, Add Rows, Remove Duplicate Rows, and Select Columns in a Data Set. These modules perform some of the most crucial data transformation tasks. As per Sarvedan data, scientists spend close to 60% of the time on data transformation. Hence, it will be particularly important to practise these modules as much as possible. Let's first learn about the Add Column modules. Let's go to the Azuraml studio for that. All right. Before we begin, upload all of the employee data sets to Azure ML Workspace. You now know how to do that. One such data set is employee data set AC 1, and the other is employee data set AC 2. I have already uploaded the AC One and AC Two data sets to Azure's ML workspace. You can download these from the Course Materials section. So now we have both the data sets, AC One and AC Two, in our saved data sets. Let's try to apply the AddColumn data transformation to them. We drag both of them onto the canvas, both AC One and AC Two. Let's drag and drop. Add columns here. Let's first search for it. All right, drag and drop it onto the canvas. As you can see, the Add Column module takes two inputs: a left data set and a right data set. To apply this transformation, all we need to do is connect the AC1 and ACT2 data sets to the Add Column module. AC one goes here and AC two goes here. And we are now ready to run this module. Before we do that, let's visualise the two data sets. The first one is an AC one. Let's visualise it. As you can see, AC One has 25 rows and eight columns. Let me close this and let's visualise AC Two. Now, AC Two has 25 rows and three columns. We expect the resultant data set to have eleven columns and 25 rows. Alright, let's see that. By running the Add Column module, it has run successfully, and let's visualise it. and it has the desired result. It has eleven columns and 25 rows, as expected. I hope it is clear by now how to merge two or more data sets and add columns. This could be very important as we deal with multiple data sets and we may need to add them or combine them vertically, that is, by columns. Let's look at how to add rows to a dataset or combine data sets using Row. sto I've already uploaded the AR1 and AR2 data sets here to save you time. Let's try to implement adding rows with these two data sets. All we need to do is drag and drop these two data sets onto the canvas. So I add AR-1 and then AR two.Now let's try to visualise the data before we apply the add rule transformation. So we click on this node and click "Visualize." As you can see, it has eleven columns and eight rows. Let's close this and go visualise the AR Two data set. It also has eleven columns but 17 rows. The columns are exactly the same as AR One. Let's close this and go back to the convo. Let's now search for "add rows" and drag and drop the "add rose" module on the canvas. Let's now provide AR One and AR Two as the input to this module by connecting these notes over here. AR One goes here, and AR Two goes to this particular note. We are now ready to run it. It has run successfully, and let's go and visualise the result data ready toWe now have 17 plus eight. That's 25 rows in the result data set. So whenever we want to merge the two data sets on rows, we can use this module to combine them for further processing. This could be important if you are dealing with some of the competitions. Because in various competitions, you may find that the training and the test data sets are provided separately. So you may want to combine them using ad rules, but not just in competition but even in day-to-day life. Various real-life data sets You may get the same information from different sources. And hence, you may want to merge those two data sets by adding rows. If it has the same number of columns and you want to process them together, let's now move on to remove duplicate rules modules. We have this employee data set here. Let's now try and visualise it. All right, as you can see, these three rows here are duplicates, and in total there are 25 rows. Even in real-life data sets, there is a possibility that you may have entire rows as duplicates. Let's now search for Remove Duplicate Rules and drag and drop it onto the canvas. Make the connections and, before we run it, we may want to launch the column selector here. It will select the columns with duplicate values, and because we want it to check if the entire row is duplicate, we are going to select all columns and press okay, and we are ready to run it now. It has run successfully, and let's visualise the output. As you can see, it has removed two duplicate values for Adam, and the number of rows is now 23 instead of the previous 25. It's a pretty straightforward module. Okay, that brings us to the last topic in this lecture, which is select columns in a data set module. This is a very important module, and we will use it in almost all our experiments. So please pay very close attention to this module. It's very simple, but because we are going to use it so often, we should pay close attention to it. Using this module, you can select only those columns that you want for further processing. There are many instances when we do not want the processor to require all columns or features from a data set. For example, IDs may not have any impact on the outcome, and hence most of the time the IDs are excluded while processing the data for any algorithm. We can use this module to select only the relevant columns. Let's understand it with an example. I have this employee data set already uploaded. Let's drag and drop it onto the canvas. Drag and drop the select columns in a data set module, make the connections, and before we run, let's select the columns we want to include in the result data set. Let's select the first four columns and press OK. There are multiple ways you can select the columns. If the requirement is to include or exclude certain types of columns or column names, you can do that here as well. You can also select columns by typing their names in this text box. As you click in this text box, it will show you all the available columns. You can also select or deselect the columns from this wizard. All right, we have these columns selected here, and let's now run this particular module. It has run successfully, and let's visualise the output. As you can see, the result dataset has only those selected columns. That completes how to select features or columns from the given data set. It also concludes the first part of data manipulation. In the next lecture, we will apply SQL transformation, and we will also edit the metadata. And finally, we will clean the missing data from the given data set. So grab yourself a cup of coffee and come to the next class. Until then, enjoy your time.
Hello and welcome to the demo course. In the last lecture, we covered how to add columns and rows, how to remove duplicate rows from a data set, as well as how to select columns in a data set. Today we are going to cover three very important topics in data transformation. Namely apply SQL transformation, how to edit the metadata, andhow to clean the missing values in a data set. These modules help us with data substitution and editing the metadata of the variables. Let's consider a scenario where you want to substitute some values for a given data set. Let's say you have a data set that has different ratings provided by the users. It could be from a survey, performance ratings, or feedback collected from various sources. But to manage and make more sense out of the data, let's say you may want to convert these values into two or three categories. Let's assume we want to categorise the ratings into low, medium, or high ratings, or any such similar skill. In such scenarios, the SQL Transformation Module allows you to modify the data using simple SQL statements. We will see some basic examples for this particular module. First, let's go to the Azure ML Studio. Here we are. I have this wine-quality dataset that I have already uploaded. Let's drag and drop the data set onto the canvas, and let's visualise it. This data set has a list of the characteristics and ingredients of wine. This dataset can be used to predict the quality of the wine. Here we have a column called Quality, which shows what the quality of the wine was for a given combination of its characteristics. We are not going to go into the details of those characteristics at this particular stage. We will see that when we actually go through and learn about classification. All right, quality has got six unique values, which are from three to eight, with three being the lowest grade and eight being the highest grade of the wine. We want to change this to, let's say, three categories: low, average, and high grid wines. Instead of the linear numeric scale, let's define three and four as low grade, five and six as average, and seven and eight as high grade. We will see how we can change that in the data set using SQL transformations. We will use a very simple SQL statement. You don't have to worry too much about the syntax, and it will add a new column called Wine Category to the existing data set. Let's search for SQL transformations. And there it is. Let's drag and drop it onto the canvas. As you can see, the SQL Transformation Module takes three datasets that are inputs and produces one output. For now, we will only use one input. I have this SQL statement, which I am going to copy and paste here to save some time. in this SQL statement. We are selecting all the columns and adding another column called Wine Category. When we use case, it will first check if the data satisfies that condition before moving to the next. In this case, we first check if the rating is less than five and then categorise that as low and less than seven. Here basically means either five or six, because it will not test that condition. If the first one is satisfied, that is for three and four. If it is also not less than seven, then we consider it high grid wine, and we call this new column "Wine Category." All right, we are now ready to run it. Let's now run this module and visualise the output afterwards. Well, it has run successfully, and let's now visualise the result. Let's go to the end of it. And there you go. It has successfully added a new column Wine Category" with three unique values, namely low, average, and high. As expected, those with prior knowledge of Escrow can try various other transformations as well. For most of the population, this code should be sufficient for typical transformations. SQL developers, please note that Azure ML does not support all the SQL statement types. So if you see that it has finished running successfully without any data in the output data set, then there are two possible reasons for that. Either no data met that requirement, or AzureML does not support that statement. The strange thing is that it will not give you any execution errors for any non-supported statements. So that's how applying SQL transformation can help you substitute values as well as perform some basic SQL data transformation. Another important module that will be required for data manipulation is called Edit Metadata. We can use this module to change the metadata that is associated with columns in a data set. There are various situations where you may want to change the data type from numeric to string or categorical, and so on. So let's try to understand that using our employee data set. Let's drag and drop it, and let's visualise it. Let's go all the way to the right. And as you can see, the column performance rating is a numeric feature and has two unique values of three and four. Assuming this is going to be in the range of one to five, we can actually make this a categorical feature. You may need to do that if you want to run a classification algorithm using the rating column as the predicted variable. And if that is the requirement, Edit Metadata will help us convert the data type from numeric to categorical. Let's see how to do that. Let's search for Edit Metadata and drag and drop it here. As you can see, it takes a data set as an input. So let's make the required connection and go through the details of editing metadata. One important point here to note thatthe data itself is not actually altered. Only the metadata inside the Azure ML tells the downstream components how to use that column. And using this module, you can change the datatypes of the columns it currently supports: string integer, floating point, boolean, date, time, and time span. You can also change the category of the feature to categorical or non-categorical, or simply change the column names by typing new names separated by commas. You can also change the field types from being a feature or label to not being a feature. The Launch Column Selector is for us to select a column whose metadata we want to change. In our case, it's the performance rating. So let's click on Launch Column Selector and select the performance rating. There you go. And let's click okay. We want to modify this performance rating to have a categorical feature so that we can run a multi-class classification on it. So we select Make Categorical and run this module. It has run successfully; let's visualise the output. Let's go to our column performance rating and select it. As you can see, it has been converted to a categorical variable or feature. I hope that clarifies how to use Edit Metadata for converting the data type. You can try it for various other options and let me know your result in the comments. Okay, so let's close this for now and let me clean this space by deleting these various modules here. The last module within the data manipulation group that we are going to cover now is called Clean Missing Data. The data that you receive will not always be ready for applying the machine learning algorithms straight away because it may have missing values. Missing values do pose a challenge in creating models for the data. Azuraml provides the Clean Missing Data module along with a few of the data cleaning methods in it. Alright, let's search for it and drag and drop it onto the canvas. We also drag and drop our employee data set and connect it to the "Clean Missing Data" as it requires an input. Let's first visualise our data set and see if it has any missing values or not. All right, in this data set we have missing values for gender and marital status. You can simply select a column and check the missing values here. All right, let's close this and go back to our Clean Missing Data module. Let's look at the parameters that are required by this particular module. This module takes five parameters. First are the columns on which we want to perform the operation. Let's launch this column selector and select the two columns where we have found missing values, that is, gender and marital status. Click okay. And another parameter that we see is the minimum missing value ratio. Here you specify the minimum number of missing values required for the operation to be performed. You should use this option in combination with the maximum missing value ratio to define the conditions under which a cleaning operation will be performed on the data set. If there are too many or too few rules that have missing values compared to the ratio you have specified, the operation will not be performed. Now, what I mean by that is that the ratio that needs to be specified should be from zero to one. 0.5 year equals 50%, and the minimum ratio is set to zero, indicating 0%, and the maximum ratio is set to one, indicating 100%. A minimum ratio of 0.1% would mean that at least 10% of the values should be missing before this transformation method can be applied. Similarly, the maximum ratio of 0.5 would meanit will only replace the missing values ofall the missing values in the data set. All right, the cleaning mode is the next parameter, and it has got multiple options for replacing or removing the missing values, with the options being "replace with Mike" for each missing value. This option assigns a new value, which is calculated by using a method described in the statistical literature as multivariate imputation using change equations. We will discuss this in greater detail in later sections as it requires some level of understanding of regression and classification. The next one is a custom substitution value. It could be zero, an A, or something else. While we do this, we have to make sure thatthe custom value is compatible with the column data type. Third one is you can replacewith mean or median or mode. We saw this term when we were talking about the basics of machine learning and some common terms. We can also replace the entire row or column that has missing values, so it would basically create a new data set from where these values would be deleted. Another option to replace or clean up missing values is using probabilistic PCA or probabilistic principal component analysis. Again, we are going to discuss this in detail in a subsequent section. Following our discussion of principal component analysis in feature selection, Let's try to replace the missing values in our data set using mode, which is nothing but the value that has the highest number of occurrences. We cannot select the mean because it is not compatible with the data type of these two columns. So if we need to process different data types and different cleaning modes, then we use different instances of this module. All right, so let's select Replace with Mode as an option, leaving all the other ones as they are, and let's run this module; it has run successfully, and let's visualise the output. As you can see, both the columns "gender" and "status" are now clean with zero missing values. All right, so that concludes the clean, missing data transformation space by deleting these various modules here. Okay, so in this lecture, we discussed how to use SQL transformations, how to edit metadata, including data types, and how to change category or feature type. And finally, we covered how to clean the missing data from the given data set. That concludes the data manipulation using Azure ML. In the next lecture, we will cover how topartition and sample the data as well as howto split the data for various requirements. Thanks. Thank you so much for joining me in this one. And I'll see you in the next class. Until then, have a great time.
Hello and welcome to this data transformation section. Today we are going to learn how to partition the data as well as split the data for training and testing purposes. There will be various instances when you may want to partition your data into multiple subsections of the same size or multiple subsections of different sizes. This could be due to various reasons, such as the input data set being too huge or having too many observations. You may want to partition the data to create, train, and test data sets. You may also want to create different sets of beanz for cross-validation of your results. You may also want to create a random sample of observations or a more balanced dataset. Let's try to understand these by going to the Azure ML studio, and let's see these modules of sample and split in greater detail. Let's begin with Partition and a sample module. So let's search for it and drag and drop it. Here we can use the partition and sample modules to perform sampling on a given data set or to create partitions from the existing data set. As you can see, it takes four parameters as input for the sampling operation, and let's try some of those using our employee data set. So there it is, and let me drag and drop this employee data set. Let's make the connections, and let's go back to our parameters. So the first parameter this module requires is partitionor sampling mode, and there are four of these. Let's try to understand and run the default one that is sampling. With this option, you can perform simple random sampling on the input data set. It will simply pick up some random rows and produce an output data set. The next one is rate of sampling, and it takes a value between zero and one. As a result, 0.1 indicates that the rows will be included in the output data set. Let's input this as 0 for 4. That is 40% of all the rows. A random seed is any integer value if we want the rows to be divided the same way every time we run it; otherwise, it will create a random sample for every run. So let this be one, two, three. Okay, and the next parameter is very important. It uses a stratified split for sampling, and it takes true or false as input. The default is false. Let's first try to understand what is meant by "stratified split" using our employee data set. For that, let's visualise the data set. All right, let's go all the way to the Performance Rating column. And as you can see, it has 20 observations rated as three and five observations rated as five. Let me close this. All right, this table simply represents the number of observations that we found in the employee data set. And in this case, if we do a random sampling with no stratification, let's say the sampling could be any subset with 40% of observations. That is ten observations of the entire data set. But what will happen is that these ten observations will be from either of these two rating values. We may get all ten observations for rating three, or five observations for rating three, and all five for rating five. Or we may get seven observations from rating Three and three observations from rating Five. Or we can get any such random combination as long as the total observations are ten, which is 40% of the data set. However, if we use stratification and provide PerformanceRating as the stratification column, the resulting data set will be very balanced. And what do I mean by that? Let's go check it out. So, with the same data set, if we set our sampling rate to 0.4% or 40% and stratify, each column value will be split in that proportion. So in our case, it will take 40% of rating three and 40% of rating five. The resultant data set will always have eight observations for rating three and two observations for rating five. Classification is very useful for categorical variables. When we apply classification, if such a data set needs to be split for training and testing, we should have a balanced and true reflection of the original data set. All right, let's go back to the Azure ML Studio. So let's use the Launch column selector to choose our Strata column. Let's select Performance Rating as our status column and click Okay. and we are ready to run it now. All right, it has run successfully, and let's visualise the output data set. As you can see, it has gotten 40 observations out of 25, which is 40% of 25%. And let's see how many observations we have for ratings three and five. We have all the values as expected. That is eight observations for rating three and two observations for rating five. I hope that clears up the concept of stratification as well as how to do sampling on a large data set. Alright, let's try another option in Sample Mode. Head is pretty straightforward and simply asks you the number of rows you want to select. You can run it with different values and visualise the output to confirm. The other two methods complement each other. So before we can pick a fold, we need to assign the rows to certain folds. You should use this option if you want to divide the data set into several subgroups. So let's first execute and assign the data to different folds. So we select this option. Select "Use Replacement" in the partitioning option. If you want certain rules to be assigned to several folds, leave it unchecked for now and select the option Randomized Split if you want rows to be randomly chosen. if you do not select this option. Rows are assigned to folds using the round-robin method. Let's type one, two, and three as the random seed value. And as you can see, the partitioner method is of two types: So either you can partition it evenly, which means it will assign an equal number of rows to each fold, or you can specify the customised proportions separated by commas. So what do I mean by that? You can specify 0.4, comma 0.2, and comma 0.4 again because we want three-partition in those particular proportions. Okay, the total of all custom values over here should always be one because if it is less than one, then it will create an additional fold with the remaining values or it may give you an error if the sum of all the proportions is greater than one. All right, let's move on to the next one. Let the stratified split remain false for now and let's run it. Great. It has run successfully, and if you try to visualise it, you will not see them as three different data sets but one single data set. I believe Microsoft needs to provide some other form of visualisation for this particular module. Anyway, let's try to visualise it using the Pick a Fold option, but for that we need another partition and sample module. So let's copy and paste this module again, and let's change the option of sample mode. To pick a fold, assign the number from which fold we want to pick up the rows. Let's say the first fold. Let's connect the nodes and run it. Well, it has run successfully, and let's visualise the output. As you can see, it has ten rows as we specified 0.4 as the proportion for the first fold. I hope you are now clear on how to use various partition and sample options, their parameters, and why it is important to partition the data. I recommend that you practise more and more on these modules because the more you practice, the more hands-on you will get and you will be more comfortable using different options once you see the effect of changing the parameters and their impact. Let's now try to understand what is meant by "Split Data Module." Let me clean the screen and drag and drop the split data module. Let's make the connections to our employee dataset, and as you can see in the properties section, split data also needs some parameters. We have already seen the last two, thatis random seed and stratified split and theywork in the exact same fashion everywhere. The fraction of rows here is the same as the sampling rate, and it assigns the fraction of rows to the first dataset and the remaining to the second data set. So if we provide zero years of observations, the first data set will contain 60% of the observations and the second one will have the remaining 40%. Let's explore the various options we have under the Split mode, split rows option. It splits the rows as perthe fraction and other parameters. We will most certainly use it for creating the train and test data sets. Recommender split is used for recommendation systems, such as if people who bought this also bought the other item. We will cover this when we learn about the AzurML recommender If we select regular expression as the splitting mode, we can specify the column name and sum beginning with text. This is useful while doing text analytics. If we want sentences starting with certain characters to be split, let's try this with employee names and those whose names start with SA and run it. It has run successfully, and if you visualise the two data sets, you will see the output as expected. Let's quickly cover the Relative Expression option, which allows us to split the data set based on some values. Let's say you want a data set with all the employees over 30 years of age, so our expression would look something like this. Okay, let's now run it and see the output. It has run successfully, and I'm going to visualise it now. As you can see, out of 25 rows, only those whose age was greater than 30 have been selected in the first dataset. Let's go back and visualise the second one. As expected, it has the remaining rows, which are less than or equal to 30. That sums up the sample and split modules from Azure ML. In this lecture, we learned about sample and split modules, and as discussed previously, we may need to use these modules if the input data set is large and has too many observations, or if you want to partition the data to create, train, and test data sets, or if you want to create different beams of data for cross-validation of your results. You can also create just a random sample of observations or a more balanced data set from the original data set. In the next and last lecture of Data Transformation, we will cover how to clip the values and how to normalise the data set. I'm sure you find it pretty easy to deal with Azure ML modules. It's all drag and drop, understanding the module, making the right connections, and you have your models getting created, which we will see in the subsequent sections. We have covered a great deal of topics here, so take a break and I'll see you in the next classroom. Until then, have a great time.
Go to testing centre with ease on our mind when you use Microsoft Data Science DP-100 vce exam dumps, practice test questions and answers. Microsoft DP-100 Designing and Implementing a Data Science Solution on Azure certification practice test questions and answers, study guide, exam dumps and video training course in vce format to help you study with ease. Prepare with confidence and study using Microsoft Data Science DP-100 exam dumps & practice test questions and answers vce from ExamCollection.
Purchase Individually
Microsoft DP-100 Video Course
Top Microsoft Certification Exams
Site Search:
SPECIAL OFFER: GET 10% OFF
Pass your Exam with ExamCollection's PREMIUM files!
SPECIAL OFFER: GET 10% OFF
Use Discount Code:
MIN10OFF
A confirmation link was sent to your e-mail.
Please check your mailbox for a message from support@examcollection.com and follow the directions.
Download Free Demo of VCE Exam Simulator
Experience Avanset VCE Exam Simulator for yourself.
Simply submit your e-mail address below to get started with our interactive software demo of your free trial.
Utilizing these DP-100 exam dumps is a good move. Moreover, it is even better if you combine them with any training course or some books. But, anyway, the usefulness of these dumps is unquestionable. They helped me pass the test easily! Use these materials with an open mind, and you will surely pass at your first attempt.
A colleague recommended me this site a while ago, and I now want to try the DP-100 practice tests from here. I hope they will help me.
I took the DP-100 exam just recently using the dumps from this site and passed! I got almost 95% of the questions right, because they were familiar for me after the practice questions. So, I can say for sure that these files are valid. Use them with some other materials, like books or lectures, and they will help you pass and earn the Microsoft Certified: Azure Data Scientist Associate certificate.
Add Comment
Feel Free to Post Your Comments About EamCollection VCE Files which Include Microsoft Data Science DP-100 Exam Dumps, Practice Test Questions & Answers.