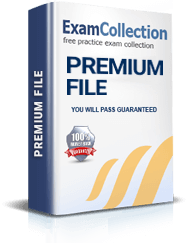
100% Real Microsoft Azure AI AI-900 Exam Questions & Answers, Accurate & Verified By IT Experts
Instant Download, Free Fast Updates, 99.6% Pass Rate
AI-900 Premium File: 232 Questions & Answers
Last Update: Feb 25, 2025
AI-900 Training Course: 85 Video Lectures
AI-900 PDF Study Guide: 391 Pages
$79.99
Microsoft Azure AI AI-900 Practice Test Questions in VCE Format
File | Votes | Size | Date |
---|---|---|---|
File Microsoft.certkiller.AI-900.v2025-01-23.by.cameron.78q.vce |
Votes 1 |
Size 1.04 MB |
Date Jan 23, 2025 |
File Microsoft.braindumps.AI-900.v2021-12-27.by.evelyn.74q.vce |
Votes 1 |
Size 793.88 KB |
Date Dec 27, 2021 |
File Microsoft.certkiller.AI-900.v2021-11-04.by.jaxon.70q.vce |
Votes 1 |
Size 830.16 KB |
Date Nov 04, 2021 |
File Microsoft.examdumps.AI-900.v2021-10-05.by.tyler.61q.vce |
Votes 1 |
Size 569.58 KB |
Date Oct 05, 2021 |
File Microsoft.test-king.AI-900.v2021-06-28.by.jaxon.53q.vce |
Votes 1 |
Size 896.22 KB |
Date Jun 28, 2021 |
File Microsoft.braindumps.AI-900.v2021-04-29.by.mohammed.47q.vce |
Votes 1 |
Size 786.62 KB |
Date Apr 28, 2021 |
File Microsoft.test4prep.AI-900.v2020-09-07.by.abdullah.25q.vce |
Votes 2 |
Size 348.57 KB |
Date Sep 07, 2020 |
Microsoft Azure AI AI-900 Practice Test Questions, Exam Dumps
Microsoft AI-900 Microsoft Azure AI Fundamentals exam dumps vce, practice test questions, study guide & video training course to study and pass quickly and easily. Microsoft AI-900 Microsoft Azure AI Fundamentals exam dumps & practice test questions and answers. You need avanset vce exam simulator in order to study the Microsoft Azure AI AI-900 certification exam dumps & Microsoft Azure AI AI-900 practice test questions in vce format.
Hi and welcome to this section on machine learning. Now, the first thing I am going to do in this section is to go out and explain a lot of the basics of machine learning. I don't want to make any assumptions for you as students that you already know about machine learning. For the benefit of those students who are not familiar with the different aspects of machine learning, I'm going to go ahead and explain these important aspects. And why do I explain these important aspects? Because it is important when you go out and implement this in Azure. The Azure machine learning service makes everything just so simple when it comes to machine learning. The most important thing for a student is to understand the basics—the different terms in machine learning. And that is what I'm going to explain at the beginning. So first I'll go through the main concepts in machine learning, and then we'll go through the machine learning service itself. We will go through the different aspects when it comes to training a machine learning model. And then we'll go and see how to deploy a machine learning model as well.
Hi and welcome back. Now, in the first set of chapters, we are going to go through some important concepts when it comes to machine learning. And the first chapter I want to go through is: What is the purpose of even considering the concept of machine learning? So for this, I want to go through a particular use-case scenario. So let's say that a company has a web application, and they have users on the Internet who are going in and accessing the web application. So let's say the web application is gettingthousands and thousands of requests per day. Assume that the company's security department wants to go ahead and analyse all of the requests that users make to the web application. They want to go ahead and analyse those requests, and they want to check if a particular request is a valid request from a user, or maybe it's a malicious request. So let's say that the security department wants to go ahead and find out if anyone is trying to go ahead and launch any sort of attack on the site from the Internet. So they want to go ahead and investigate whether any malicious requests are being made to that web application. So, in order to actually get this information, in order to decipher this information, they would go ahead and take various details of the requests that are being made. So they might be logging this request on the server that is hosting the Web application. So over here they might take details such as the type of request that has been made, the source IP address of the request that is being made, and let's say how many requests are coming in per second, taking all of this information along with other information that might be present. They would then go ahead and try to understand whether the request that they made is a valid request or whether it's a malicious request. This is based on various parameters. Now, they could actually go ahead and do this with the help of a program. So maybe they might have a development team that could go ahead and develop a programme or a script. It will take all of this information about the various requests and then determine whether or not each request is valid or malicious. But again, there could be a lot of timethat could be taken in order to go aheadand develop this programme or develop this script. Also, in today's world, there can be different sorts of ways to go ahead and actually launch attacks on a web application. So this keeps on changing. So if the data itself is changing, let's say that there is more information that needs to be obtained in order to go ahead and decide whether a request is valid or not. Then they might need to go ahead and change the programme itself or the script itself. So in this case, You can actually go ahead and make use of machine learning instead of actually going in and developing that programme or writing that script. You can actually go ahead and make use of machine learning and anomaly detection to go ahead and just take the data. And machine learning itself could go ahead and give you that information as to whether a request is valid or not. So instead of actually going ahead and developing that programme or the script, you can actually go ahead and make use of machine learning algorithms to go out and train something known as a machine learning model. That machine-learning model can actually go ahead and take your data and then go ahead and give you sort of an indication as to whether a request is valid or not. So in this chapter, I just want to kind of start off with the purpose of machine learning to give you an idea of a use-case scenario where you can actually go ahead and make use of machine learning.
Now in this chapter, I want to go through the concept of the machine learning model. So when it comes to machine learning, let's say you want to go ahead and use machine learning to give you a prediction. Consider the following use-case scenario: suppose you want to predict whether a person will develop diabetes. Let's say that you have the medical history information for a lot of people. You have a lot of data in place. And based on that historical data, you already know whether a person might develop diabetes or not. And now, using that historical data, you want to ensure that you can make future predictions for people on whether they will develop diabetes or not if they feed in their medical information, their medical history information. So over here, you are trying to build something known as a machine learning model. That machine learning model is nothing but a function. That function takes in your input values, that is, x, just for simplicity. So over here, you might feed in the different medical information about a person onto that machine learning model, onto that function, and then it'll go ahead and give you an output value. That output value is your prediction of whether a person would actually develop diabetes based on the medical information you have provided about that particular person. So over here, the main thing is to go ahead and develop your function, so you have your input data and you know what you want to get out of that machine learning model. So over here, you have to go ahead and develop that machine learning model. You have to go ahead and develop that function. So I say the entire purpose of machine learning is that you should not be the one to go ahead and develop that function. So you should not go ahead and have a Dolphin team go ahead and write a function, write a program, develop a script, and then go ahead and take that information and give you an output. The entire purpose of machine learning is to go ahead and take in data for which you already know whether a person will develop diabetes or not. And then machine learning itself will go ahead and learn from that data and understand or develop a model. And then you can go ahead and use that modelto go ahead and depict in the future, predict inthe future whether a person would develop diabetes or not. So, in this case, when it comes to machine learning, the model is what you will create in order to make future predictions. Now, in order to go ahead and develop a machine learning model, you'll actually go ahead and make use of existing machine learning algorithms. So algorithms are just a way to help build that machine learning model. Now, there are already many machine learning algorithms that have already been defined. So the algorithm basically tells machine learning what you should try to do to develop that model based on the data that you provide. Again, I'll go through a little bit of depth when it comes to machine-learning algorithms a little bit later on. But what you do is, when you want to go ahead and develop a machine learning model, you'll go ahead and feed in some data. You'll also go ahead and feed in the machine learning algorithm. And then the machine learning process will actually go ahead and develop that model or that function based on the data that you provide and based on the machine learning algorithm. So now that we have this concept in place, let's go on to the bit about training your model. So I said that the first thing that you have to do is go ahead and build your model. You have to go ahead and build the function based on the data and based on the algorithm. So that building of the model is known as "training." You are training your model to go ahead and try to understand, let's say, the case of predicting diabetes for a particular person. So that model should be able to be trained and then decide that if in the future you do provide information about the medical history of a person, it should be able to make the right prediction as to whether a person will develop diabetes or not. So you have to go in and train the model. Training the model is basically building your function. Now how do you train the model? So you train the model based on the algorithm. That algorithm will then work on some test data that you will provide. So the data in this case is actually known as training data. So if I just go ahead and scroll down again, don't worry. So we'll be going through some use-case scenarios a little bit later on, and then all these concepts will actually make much more sense if you don't really understand them right now. So I don't want to give you an introduction to the different concepts. First is the machine learning model; then is the machine learning algorithm. And now I'm talking about the data. So in order to go ahead and train your model, you have to go ahead and feed data into the model. Because, over here, we are not developing a function. We want machine learning to go ahead and give us the function based on the data that we provide. and this data is known as training data. So we'll have a data set in place. So let's say I said when it comes to diabetes, we might have information, medical information, about people who have already gone ahead and developed diabetes or not, and you might have different information about these different patients. For example, it could be the blood sugar levels, etc. For and then over there you'll have a columnas to whether the person has diabetes or not. So you'll go ahead and take that information; you'll go out and give it to the machine-learning process. That machine learning process will go ahead and take that data, use the machine learning algorithm, and then try to create that machine learning model or function. Now, normally, when you have a data set in place, you'll actually go ahead and take some of the data to go ahead and train the model, and then you'll go ahead and take a part of that data to test the model. So let's say machine learning has gone ahead and created the model for you based on the data that you have provided. Now, in order to go ahead and understand whether the model is accurate or not, because that is something that you need to do, especially when it comes to, let's say, the medical field, If you want to go in and determine, as I said in this case, the diabetes of a particular person, you want to make a prediction about whether that person will develop diabetes or not. It's very important that your model be accurate, and the function should be accurate. So you have to go ahead and test the model. So when you have your data in place, what you're supposed to do is to go ahead and take that data and basically split it into data that can be used to train the model and data that can be used to test the model. So normally, 70% of your data is used to train the model, and 30% of your data is used to go out and test the model. So based on this data that you provide, you'll have a good machine learning model in place, and this is always an iterative process. So let's say you have a machine learning model in place; it's going in and making predictions for you. Let's say that no data changes. So, let's say that in order to predict whether a person will develop diabetes or not, there is a standard that must be followed in order to make this decision. So then you have to go ahead and retrain your model with the new data. So this is always an iterative process becausethe machine learning model can or it's verydifficult to go ahead and be 100% accurate. It is your responsibility to ensure that you keep on training a model to go and at least achieve close to 100% accuracy when it comes to, let's say, making a prediction, right? So in this chapter, I just want to kind of go through this important information when it comes to the machine learning model and when it comes to the data for the machine learning model.
Hi and welcome back. Now in this chapter, I just want to give a brief overview of machine learning algorithms. So when it comes to creating your machine learning model, you go ahead and feed in two things to the machine learning process. First is your data, and the other is your machine learning algorithm. So the algorithm is basically going to tell machine learning how to go ahead and process the data and then develop an efficient machine learning model to go ahead and develop that function. Now there are many machine-learning algorithms that are already in place. So over here in the Microsoft documentation, when it comes to machine learning, over here they're going and giving examples of different machine learning algorithms, and I'll just go ahead and give a brief overview when it comes to one of them, and that is linear regression. So, in the end, that's what the algorithm tries to do, which is why I suggested feeding your data into the machine learning process. Now let's say over here you have these differentdata points that have been plotted on a graphbased on the data that you have provided. Now it is the job of the machine learning algorithm to go ahead and try to find out the relationship between the data values that you have plotted. So over here in terms of linear regression, it's trying to go ahead and just find a linear relationship between the values that have been plotted on the graph. If you have more complex data values, then you would probably have to go ahead and look at other machine learning algorithms. So based on what you are trying to achieve and what you are trying to predict based on the data itself, you have to go ahead and decide what machine learning algorithm you need to use. Now, again, there's a lot of math and statistics that are actually behind the creation of a machine learning algorithm. And definitely, when it comes to the perspective of this exam, you don't have to go ahead and understand in depth in terms of what goes into building a machine learning algorithm because that's already in place. There are all these standard-defined machine learning algorithms. The job of a data scientist is actually to go ahead and see how to make effective use of those algorithms to go ahead and create something known as a machine learning model. And definitely in this section we are going to go ahead and see how to build a machine learning model in Azure Machine Learning Studio, right? So these are the different algorithms that you have in place. So let me go ahead and give you kind of a brief example of the linear regression algorithm. So I said in the end that when you look at the machine learning model, it's nothing but trying to find the relationship between the output and the input using a function. So when it comes to a linear regression algorithm, it's trying to find the relationship between the response and one or more variables. So over here, Y is your response, and X is your set of variables of data. Now, you could go ahead and feed in multiple variables of data if you just have one input variable. It's known as simple linear regression. And if you have multiple input variables, it is known as multiple linear regression. Now, over here, just as an example when it comes to linear regression, let's say I have this data in place wherein I have orders that have been placed by customers. Assume there is a site that publishes courses, and these are the number of courses purchased during a specific R in a day. So over here, in terms of the Rs, I'm going to give a numerical representation. So, let's say you want to make a future prediction based on this data about the number of orders that could be placed the following day. Now, obviously, in order to go ahead and make a very useful prediction, you will actually go ahead and feed in a lot of data, and not just data for just one day. You might want to go ahead and populate the orders that are made probably per minute in a day or actually submit orders that have been placed over the course of a week or a month in order to create a good machine learning model. But just to give you an explanation, when it comes to at least an overview, when it comes to the linear regression algorithm, I'm trying to keep this as simple as possible. So over here, we are trying to go ahead and use the data that we have to go ahead and make a prediction in terms of the orders. So over here, when it comes to our function, y is equal to the function of x. So over here, remember, we're trying to go ahead and determine our function. So over here, x is nothing but our input information, and why is the order that we are going to try and predict. Now, when it comes to linear regression So the first thing it is going to do is go ahead and basically plot this graph. So I've got the graph down as well. So over here in terms of the graph, we have our order information. So over here we have the Rs in the day, and over here we have the number of orders. Now, based on this data, the linear regression algorithm is going to try and plot a line to go ahead and determine what the relationship is between the hours in a day and the number of orders that are being made. So for example, on a particular day, if you wanted to go ahead and find out, okay, let's say at 10:00, what was the number of orders that we might receive, you could go ahead and find the orders based on the line that was being plotted, giving a very simplistic example. So how does it actually go ahead and plot this line? So first it will go ahead and plot a random line. Then it will go ahead and see how many data points are on this side of the line and on this side of the line to try and understand whether it's plotting the right line when it comes to the relationship. If not, it will now try to go ahead and plot two additional lines on both sides and see if either of these lines is again accurate when it comes to determining the relationship between the X and the Y values, and so on and so forth. Now, that is one point. So once it has gone ahead and determined where the line should be over here, it then starts to go ahead and determine where the line should start from. So over here, it's not necessary that the line should start from zero itself. So over here, the algorithm is trying to figure out what is the best way to go ahead and define the relationship between the data values, and this relationship will actually form the basis of your function, and this will form the basis of your machine learning model. So over here, I just want to kind of give you an idea when it comes to the purpose of the machine-learning algorithm, using a pretty simple example.
Hi and welcome back. Now in this chapter, I just want to go through the different types of machine learning algorithms that are in place. So there are already different machine learning algorithms that are already defined. So these are based on different types. So for example, I already talked about the regression algorithms. So this can be used to go ahead and predict the value of a new data point based on historical data. So that's something that we already know. Now, there are other types of algorithms as well. So first, you have classification algorithms. So over here, you are just trying to go ahead and assign a category to new data values. So you have the two-class binary classification algorithm, wherein you just have a simple answer that you want to go ahead and predict. So for example, you might want to go ahead and predict what the stock price will be tomorrow. Over here, you just have a simple answer, whether it's a yes or a no. So you go out and feed it along with the algorithm to go ahead and build your model based on previous stock prices and basically the label data that you have, whether the stock price goes up or down. And you only want to go ahead and predict at this point in time whether the stock price will go up or down. You also have multi-class classification algorithms. So over here, I'm just giving an example. So over here, which ticket class might the customer purchase? Either it could be an economy ticket, a business ticket, or a first-class ticket. So over here, you're trying to classify the data into different labels. Then you have anomaly detection algorithms. So you're trying to find or identify data points that are outside of the defined parameters of what is considered normal. So for example, when it comes to banks, they want to go ahead and find out if a credit card purchase is a fraudulent purchase or not. This is an example of anomaly detection. So you have algorithms in place for this house. Well, then you have time series algorithms. So here, you want to go ahead and see how values change over time. And this is when it comes to predicting, as an example, stock prices in the future. And then you have clustering algorithms that divide data into multiple groups. So over here, you're trying to look at the similarity of data points. So an example is, what types of food do customers like to order? So in this chapter, we'll kind of go through the different types of machine learning algorithms that are out there.
Go to testing centre with ease on our mind when you use Microsoft Azure AI AI-900 vce exam dumps, practice test questions and answers. Microsoft AI-900 Microsoft Azure AI Fundamentals certification practice test questions and answers, study guide, exam dumps and video training course in vce format to help you study with ease. Prepare with confidence and study using Microsoft Azure AI AI-900 exam dumps & practice test questions and answers vce from ExamCollection.
Purchase Individually
Microsoft AI-900 Video Course
Top Microsoft Certification Exams
Site Search:
SPECIAL OFFER: GET 10% OFF
Pass your Exam with ExamCollection's PREMIUM files!
SPECIAL OFFER: GET 10% OFF
Use Discount Code:
MIN10OFF
A confirmation link was sent to your e-mail.
Please check your mailbox for a message from support@examcollection.com and follow the directions.
Download Free Demo of VCE Exam Simulator
Experience Avanset VCE Exam Simulator for yourself.
Simply submit your e-mail address below to get started with our interactive software demo of your free trial.
Good Morning ,I am kindly requesting If they are new updated AI-900 as I am writing on 4th March 2022 ?
The exam was updated on 27 January. Are there any dumps most recent than September 2020 available?
I am planning to attempt AI-900 exam
Need AI-900 certification dump
Correct dump. Pass exam with 920.
Add Comment
Feel Free to Post Your Comments About EamCollection VCE Files which Include Microsoft Azure AI AI-900 Exam Dumps, Practice Test Questions & Answers.